14:15 |
0072.
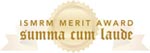 |
A frame work for non-rigid
motion corrected compressed sensing for highly accelerated
MRI 
Muhammad Usman1, Christoph kolbitsch1,
Ghislain Vaillant1, David Atkinson2,
Tobias Schaeffter1, Philip G. Batchelor1,
and Claudia Prieto1,3
1King's College London, London, United
Kingdom, 2University
College London, 3Escuela
de Ingenieria, Pontificia Universidad Catolica de Chile,
Santiago, Chile
Motion during MRI acquisition can cause inconsistencies
in k-space, resulting in strong artefacts in the
reconstructed images. Accelerated imaging using
compressed sensing (CS) requires motion correction
approaches not just to correct the motion related
artefacts, but also to retain the sparsity level in the
sparse representation, which is one of the requirements
of CS reconstruction. Currently, only translational
motion correction methods have been combined with CS. In
this work, we propose a novel Motion
Correction-Compressed Sensing (MC-CS )technique that can
correct for any arbitrary non-rigid motion in CS
undersample acquisitions. This approach was tested both
in simulations and in-vivo data for 2D CINE MRI, and use
to reconstruct respiratory motion-free cardiac cycle
from free-breathing acquisitions.
|
14:27 |
0073. |
Three-Dimensional
Hybrid-Encoding for Compressed Sensing MRI 
Haifeng Wang1, Dong Liang2, King F
Kevin3, Gajanan Nagarsekar1, and
Leslie Ying1
1Department of Electrical Engineering and
Computer Science, University of Wisconsin-Milwaukee,
Milwaukee, WI, United States, 2Shenzhen
Institutes of Advanced Technology, Chinese Academy of
Sciences, Shenzhen, Guangdong, China, 3Global
Applied Science Laboratory, GE Healthcare, Waukesha, WI,
United States
In compressed sensing with Fourier encoding, the low
spatial frequency always has to be fully sampled such
that the high frequency is insufficiently sampled at
high accelerations, leading to serious loss of
resolution. In this paper, we propose a novel 3-D
acquisition method using hybrid encoding. The method
exploits random encoding with a circulant structure
along one direction, while keeping conventional Fourier
encoding along the other two directions. Both simulation
and experimental results demonstrate that the proposed
method preserves better resolution than the conventional
3-D Fourier encoding when the same acceleration factor
is used.In compressed sensing with Fourier encoding, the
low spatial frequency always has to be fully sampled
such that the high frequency is insufficiently sampled
at high accelerations, leading to serious loss of
resolution. In this paper, we propose a novel 3-D
acquisition method using hybrid encoding. The method
exploits random encoding with a circulant structure
along one direction, while keeping conventional Fourier
encoding along the other two directions. Both simulation
and experimental results demonstrate that the proposed
method preserves better resolution than the conventional
3-D Fourier encoding when the same acceleration factor
is used.In compressed sensing with Fourier encoding, the
low spatial frequency always has to be fully sampled
such that the high frequency is insufficiently sampled
at high accelerations, leading to serious loss of
resolution. In this paper, we propose a novel 3-D
acquisition method using hybrid encoding. The method
exploits random encoding with a circulant structure
along one direction, while keeping conventional Fourier
encoding along the other two directions. Both simulation
and experimental results demonstrate that the proposed
method preserves better resolution than the conventional
3-D Fourier encoding when the same acceleration factor
is used.
|
14:39 |
0074. |
Parameter-Free Compressed
Sensing Reconstruction using Statistical Non-Local
Self-Similarity Filtering 
Mariya Doneva1, Tim Nielsen1, and
Peter Börnert1
1Philips Research Europe, Hamburg, Germany
In this work, we present a CS reconstruction based on
statistical non-local self-similarity filtering (STAINLeSS).
The method provides improved image quality compared to
wavelet based CS reconstruction and does not require any
parameter adjustments. All the parameters are
automatically determined by the noise estimation in the
receive channels obtained from a standard noise
measurement.
|
14:51 |
0075. |
Joint Bayesian Compressed
Sensing with Prior Estimate 
Berkin Bilgic1, and Elfar Adalsteinsson1,2
1EECS, Massachusetts Institute of Technology,
Cambridge, MA, United States, 2Harvard-MIT
Division of Health Sciences and Technology, MIT,
Cambridge, MA, United States
In clinical MRI, it is routine to acquire images with
different contrasts for increased diagnostic power. Yet
depending on the imaging sequences, acquiring certain
contrasts is relatively faster. Here, a Bayesian
compressed sensing (CS) algorithm that uses a
fully-sampled image as prior information to help
reconstruct images from undersampled k-space is
presented. This method substantially improves the
reconstruction quality, and allows joint reconstruction
of multi-contrast images.
|
15:03 |
0076. |
Dynamic Imaging Using
Sparse Sampling with Rank and Group Sparsity Constraints 
Anthony G. Christodoulou1,2, S. Derin Babacan2,
and Zhi-Pei Liang1,2
1Department of Electrical and Computer
Engineering, University of Illinois at Urbana-Champaign,
Urbana, IL, United States, 2Beckman
Institute of Advanced Science and Technology, University
of Illinois at Urbana-Champaign, Urbana, IL, United
States
This work highlights a novel dynamic imaging method
which jointly uses partial separability (PS), sparsity,
and group sparsity constraints to enable sparse sampling
in (k,t)-space. The specific formulation of the group
sparsity spatially varies the effective model order of
the PS constraint as a form of controlling the balance
between the PS and sparsity constraints.
|
15:15 |
0077.
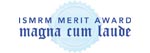 |
Blind Compressed Sensing
Dynamic MRI 
Sajan Goud Lingala1, and Mathews Jacob2
1Biomedical Engineering, The University of
Iowa, Iowa city, IA, United States, 2Electrical
and Computer Engineering, The University of Iowa, IA,
United States
In this work, we introduce a novel blind compressive
sensing frame work for dynamic MRI reconstruction. This
models the temporal profile at each voxel as a sparse
linear combination of temporal basis functions chosen
from a large dictionary, which are also estimated from
the data. We show that the model significantly reduces
the number of degrees of freedom than what is seen in
schemes based on promoting low rank structure of the
data. We demonstrate this concept on myocardial
perfusion data sets with significant inter-frame motion.
Significant improvement in the reconstruction qualities
over low rank schemes are observed (eg: better
preservation of subtle spatial details, reduced temporal
blur and artifacts).
|
15:27 |
0078. |
MMSE optimal non-local
motion compensation for compressed sensing cardiac cine
imaging using k-t FOCUSS 
Huisu Yoon1, and Jong Chul Ye1
1Bio and Brain engineering, KAIST, Dae-jeon,
Korea, Republic of
Compressed sensing (CS) tells us that the perfect
reconstruction is possible if the nonzero support in
transform domain is sparse and sampling basis are
incoherent. By exploiting that dynamic MRI can be
sparsified due to the temporal redundancy, we have
demonstrated successful application of CS for cardiac
imaging. In particular, more accurate prediction using
motion estimation/compensation or data-driven optimal
temporal sparsifying transforms have proven to be quite
effective. However, despite their successes to some
extent, there still remain considerable artifacts in
edge area when the acceleration factor increases.We
propose a non-local motion compensated k-t FOCUSS which
generates more accurate prediction images than the
existing motion compensated k-t FOCUSS. Non-local motion
compensation retrieves similar blocks in another
reference frame, not within the processed dynamic frame
itself. Non-local motion compensation is shown MMSE
optimal and experimental result shows that the proposed
algorithm clearly reconstruct the important cardiac
structures and improves over k-t FOCUSS.
|
15:39 |
0079. |
Spatial and Temporal
Behaviors in Rapid DCE MRI with and without Compressed
Sensing 
Kyunghyun Sung1, Manoj Saranathan1,
Brady Quist1,2, Shreyas S Vasanawala1,
Bruce L Daniel1, and Brian A Hargreaves1
1Radiology, Stanford University, Stanford,
CA, United States, 2Electrical
Engineering, Stanford University, Stanford, CA, United
States
High spatial and temporal resolution is desirable for
many dynamic contrast enhanced (DCE) MRI applications.
Many view sharing methods have been developed to improve
both spatial and temporal resolution, but those methods
inherently increase temporal footprints, resulting in
temporal blurring. In this work, we show a temporal
footprint of the view sharing method can be improved by
reconstructing the individual subsampled k-space using a
novel CS reconstruction while maintaining excellent
image quality in a total of 12 DCE MRI patients.
|
15:51 |
0080. |
Reconstruction for Dynamic
2D-Radial Cardiac MRI Using Prior Enhanced Compressed
Sensing 
Ti-chiun Chang1, Mariappan S. Nadar1,
Jens Guehring2, Michael O. Zenge2,
Kai T. Block3, Peter Speier2,
Michael S. Hansen4, and Edgar Mueller2
1Siemens Corporate Research, Princeton, NJ,
United States, 2Siemens
AG, Erlangen, Germany, 3NYU
Langone Medical Center, New York, NY, United States,4National
Institutes of Health, Bethesda, MD, United States
Compressed sensing (CS) theory emerges as a promising
approach that can accurately reconstruct a signal f even
when its indirect measurement is severely undersampled.
In the basic CS framework, the sparsity is the only
prior knowledge exploited. In practice, measurement
imperfections and noise are present, so the data
reduction factor promised by CS is clearly reduced. To
achieve better results, effort has been devoted to
incorporating more prior estimates. In this work, the
reconstruction results for dynamic 2d radial cardiac MRI
are improved by using additional prior obtained from
combining the interleaved samples in the dynamic image
sequence
|
16:03 |
0081. |
K-t Radial SPARSE-SENSE:
Combination of Compressed Sensing and Parallel Imaging with
Golden Angle Radial Sampling for Highly Accelerated
Volumetric Dynamic MRI 
Li Feng1,2, Hersh Chandarana1,
Jian Xu3, Kai Tobias Block1,
Daniel Sodickson1, and Ricardo Otazo1
1Radiology, New York University School of
Medicine, New York, New York, United States, 2Sackler
Institute of Graduate Biomedical Sciences, New York
University School of Medicine, New York, New York,
United States, 3Siemens
Medical Solutions, New York, New York, United States
Radial k-space sampling is a good candidate for
compressed sensing due to the inherent incoherent
aliasing artifacts. For dynamic applications, the
incoherence can be further increased by using the
golden-angle approach, which completely avoids
acquisition of replicate radial lines. Moreover, the
golden-angle approach allows for reconstruction of
arbitrary time points with arbitrary temporal
resolution. In this study, we propose a joint
non-Cartesian image-reconstruction technique that
combines compressed sensing and parallel imaging for
accelerating volumetric dynamic MRI with stack-of-stars
golden-angle radial trajectories. The performance of
this technique is demonstrated for highly accelerated 3D
free breathing liver perfusion imaging.
|
|