10:30 |
0686.
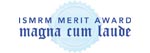 |
Brain network metric derived
from DWI: application to the limbic system 
Luis Manuel Colon-Perez1, Caitlin Spindler2,
Shelby Goicochea3, William Triplett4,
Mansi Parekh5, Eric Montie6, Paul
Richard Carney5,7, and Thomas Mareci4
1Physics, University of Florida, Gainesville,
Florida, United States, 2Biology,
University of Florida, 3Chemistry,
University of Florida, 4Biochemistry
& Molecular Biology, University of Florida, 5Pediatrics,
University of Florida, 6Science
and Mathematics, University of South Carolina Beaufort,
Bluffton, South Carolina, United States, 7Wilder
Center of Excellence for Epilepsy Research, University
of Florida
MRI derived measurements such as brain network measures
are inherently discrete and are affected by the
resolution of acquisition. Appropriate normalization
techniques permit the use of DWI and tractography
methods to create measurements that would allow the
study of the fibrous structure within the brain. Here we
present an edge weight metric used to define the
connectivity strength of a network node that would allow
a better understanding of local networks in the brain.
With this edge weight metric, anatomical structures are
defined, i.e. nodes, and the implied white matter tracts
from tractography give rise to the edge.
|
10:42 |
0687. |
The effect of tractography
algorithm on human cortical connectome reconstruction by
diffusion weighted MRI 
Matteo Bastiani1,2, Rainer Goebel1,
and Alard Roebroeck1
1Department of Cognitive Neuroscience,
Faculty of Psychology and Neuroscience, Maastricht
University, Maastricht, Netherlands, 2Forschungszentrum
Jülich, Jülich, Northreinwestfalen, Germany
This work focuses on reconstructing the macroscopic
human cortical connectome using diffusion weighted
imaging. This can be represented as a graph where
cortical patches are nodes and white-matter connections
are edges. The effects on estimating the most common
graph measures when using single versus multi direction
diffusion models, deterministic versus probabilistic
tractography, and local versus global measure-of-fit of
the reconstructed fiber trajectories are evaluated. We
show that these choices, together with anisotropy,
curvature and probabilistic threshold parameters can
strongly affect connection density, small-worldness and
cortical connection hubs. This is an important
consideration for studies using these measures as
dependent variables.
|
10:54 |
0688. |
Dendrogram processing for
whole-brain connectivity-based hierarchical parcellation 
David Moreno-Dominguez1, Alfred Anwander1,
and Thomas R Knösche1
1Max Planck Institute for Human Cognitive and
Brain Sciences, Leipzig, Germany
Hierarchical clustering of probabilistic tractograms
encodes the information of the connectivity structure at
all granularity levels in a hierarchical tree or
dendrogram. It might be the key to whole-brain
connectivity based parcellation, where the correct
number of clusters is unknown and depends on the desired
granularity. The interpretation of the resulting
dendrogram is not simple, due to outliers and the size
of the dataset encoded, among other reasons. In this
study a fast, fully hierarchical bottom-up algorithm is
presented, and intelligent processing steps are
introduced in order to ease the information extraction
process, successfully enabling better performance of
tree-partitioning algorithms.
|
11:06 |
0689.
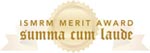 |
SIFT: Spherical-deconvolution
Informed Filtering of Tractograms 
Robert Elton Smith1,2, Jacques-Donald
Tournier1,2, Fernando Calamante1,2,
and Alan Connelly1,2
1Brain Research Institute, Florey
Neuroscience Institutes, Heidelberg, Victoria,
Australia, 2Department
of Medicine, University of Melbourne, Melbourne,
Victoria, Australia
When performing whole-brain fibre-tracking using
Diffusion-Weighted Imaging, certain pathways in the
brain can be over- or under-estimated, due to the
independence of individual streamlines, and any
methodological biases of the models or algorithms used.
To improve the biological accuracy of connectome
reconstruction, we propose a normalisation process for
whole-brain fibre-tracking data, which uses the results
from spherical deconvolution to selectively filter
tracks from the data set, matching the track densities
in high angular resolution space to the estimated
intra-cellular volume fractions.
|
11:18 |
0690. |
Comparison of Fiber
Tractography Based on Susceptibility Tensor Imaging and
Diffusion Tensor Imaging 
Chunlei Liu1,2, Bing Wu1, and Wei
Li1
1Brain Imaging and Analysis Center, Duke
University, Durham, NC, United States, 2Department
of Radiology, Duke University, Durham, NC, United States
A method of fiber tractography based on STI was
demonstrated. STI experiments of perfusion-fixed mouse
brains were conducted and magnetic susceptibility
tensors were calculated for each voxel with
regularization and decomposed into its eigensystem. The
major eigenvector was found to be aligned with the
underlying fiber orientation. Following the orientation
of the major eigenvector, we were able to map
distinctive fiber pathways in 3D. As a comparison,
diffusion tensor imaging (DTI) and DTI fiber
tractography were also conducted on the same specimens.
The relationship between STI and DTI fiber tracts was
explored with similarities and differences identified.
|
11:30 |
0691.
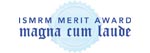 |
Comparison of probabilistic
diffusion tensor tractography with histological tracer
studies and RSFC in the rhesus macaque 
Elizabeth Zakszewski1, Andrew S Fox1,
Jonathan Oler1, Nagesh Adluru1,
Alexander K Converse1, Jeffrey M Moirano1,
Ned Kalin1, and Andrew L Alexander1
1University of Wisconsin, Madison, WI, United
States
Examinations of probabilistic tractography have
identified major tracts across the brain, but little has
been done to compare them to well-established tracer
studies. Therefore, diffusion tensor imaging (DTI)
probabilistic tractography of white matter (WM) was
performed on a DTI template generated from 271 rhesus
macaques. Injection sites from tracer experiments in
literature were chosen as seed regions from which to
begin tractography. Results demonstrated the
probabilistic connectivity maps obtained on the DTI
template were largely consistent with connectivity maps
from the tracer studies.
|
11:42 |
0692. |
Distinct Components of the
Cingulum Bundle Revealed by Diffusion MRI 
Derek K Jones1, Kat F Christiansen1,
Rosanna J Chapman1, and John P Aggleton1
1CUBRIC, School of Psychology, Cardiff
University, Cardiff, Wales, United Kingdom
The cingulum bundle is a complex tract comprised of many
different connections with trajectories of different
lengths. However, this complex composition is rarely
reflected in published diffusion tractography images,
which most often show a continuous band of white matter
that seemingly links (uninterrupted) the caudal medial
temporal lobe with retrosplenial, cingulate, prefrontal,
and subgenual areas. This work examined the unity of the
cingulum bundle using tractography by comparing results
from ROIs at different points along the tract and
reveals a set of overlapping, but largely separate,
connections within what is often visualised as a unified
bundle.
|
11:54 |
0693. |
Whole-brain DSI in 4
minutes: sparse sampling in q-space with simultaneous
multi-slice acquisition 
Kawin setsompop1, Berkin Bilgic2,
Julien Cohen-Adad1, M. Dylan Tisdall1,
Boris Keil1, Thomas Witzel1,
Yogesh Rathi3, Van J Wedeen1,4,
Elfar Adalsteinsson2,4, and Lawrence L Wald1,4
1Dept. of Radiology, A. A. Martinos Center
for Biomedical Imaging, MGH, Charlestown, Massachusetts,
United States, 2EECS,
Massachusetts Institute of Technology, Cambridge,
Massachusetts, 3Brigham
and Women's Hospital, Boston, Massachusetts, United
States, 4Harvard-MIT
Division of Health Sciences and Technology
In this work, we demonstrate an improvement in the time
efficiency of DSI acquisitions using three complementary
technologies: (i) high-strength gradient coils, (ii) a
Simultaneous Multi-Slice (SMS) acquisition with Blipped-CAIPI
readout using a highly parallel receive coil array, and
(iii) a compressed sensing reconstruction that enables
undersampling of q-space. Together, these improvements
allow for an acquisition of high-quality whole-brain DSI
data in just over 4 minutes. While this initial
demonstration focuses on DSI, the general approach
should be applicable to other HARDI acquisition schemes.
|
12:06 |
0694.
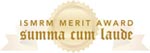 |
Improved Q-Ball imaging
using a 300 mT/m human gradient 
Julien Cohen-Adad1, M Dylan Tisdall1,
Ralph Kimmlingen2, Eva Eberlein2,
Thomas Witzel1, Philipp Hoecht2,
Boris Keil1, Juergen Nistler2,
Dietmar Lehne2, Keith Heberlein3,
Jennifer A McNab1, Herbert Thein2,
Franz Schmitt2, Bruce R Rosen1,
Van J Wedeen1, and Lawrence L Wald1,4
1A. A. Martinos Center for Biomedical
Imaging, Massachusetts General Hospital, Harvard Medical
School, Charlestown, MA, United States, 2Siemens
Healthcare, Erlangen, Germany, 3Siemens
Healthcare, Boston, United States, 4Harvard-MIT
Division of Health Sciences and Technology, MIT,
Cambridge, MA, United States
In this work we used a novel Connectom Gradient (AS302)
system specifically designed to achieve ultra-high
gradient strength (Gmax = 300 mT/m , about 7.5 fold
stronger than clinical scanners). This enables to
shorten the diffusion-encoding time and thereby decrease
the TE, yielding significant gains in SNR. In addition,
we developed a 64-channel receive coil to further
increase the SNR. We compare the in vivo HARDI data with
Q-Ball reconstruction at variable gradient strengths and
b-values up to 15000 s/mm2 in human. The higher SNR is
shown to provide improvements in ODF metrics such as the
fiber uncertainty and FA.
|
12:18 |
0695.
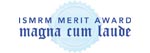 |
A new multi-directional
fiber model for low angular resolution diffusion imaging
permission withheld
Aymeric Stamm1, Patrick Pérez2,
and Christian Barillot1
1Visages INSERM/INRIA U746, IRISA - UMR CNRS
6074, Rennes, France, 2Technicolor,
Rennes, France
Clinical brain diffusion imaging provides low angular
resolution diffusion images that were up to now analyzed
under the assumption of Gaussian diffusion. An important
limitation of such an analysis is that it does not
exhibit crossing fibers. In this abstract, a
non-Gaussian diffusion profile is assumed that allows
for the accurate estimation of crossing fibers from low
angular resolution diffusion images.
|
|