16:00 |
0407. |
Advancing the clinical
utility of MRI with online quantitative analysis:
application to brain tissue classification in multiple
sclerosis 
Refaat E Gabt1, Xiaojun Sun1, Amol
Pednekar2, and Ponnada A Narayana1
1Diagnostic and Interventional Radiology,
University of Texas Health Science Center at Houston,
Houston, TX, United States, 2Philips
Healthcare, Cleveland, OH, United States
The quantitative power of MRI in neuroimaging is largely
limited to research purposes and hardly finds its way
into the clinical world, primarily due to the
computationally-intensive nature of quantitative
neuroimaging and the large amount of MRI data. We
developed an integrated image acquisition and analysis
pipeline that seamlessly combines the data acquisition
with the high performance computation. To demonstrate
the power of this pipeline, in 16 multiple sclerosis
patients, the brain was classified into white matter,
grey matter, cerebrospinal fluid, and white matter
lesions in under one minute. The tissue maps were
imported back to the scanner for clinical review.
|
16:12 |
0408.
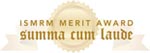 |
Cross-scale self-similarity
super-resolution of single MRI slice-stacks 
Esben Plenge1 and
Michael Elad1
1Dept. of Computer Science, Technion - Israel
Institute of Technology, Haifa, Israel
A new method for super-resolution reconstruction of
single MRI slice-stacks is presented. It exploits the
fact that both high-resolution and low-resolution image
content is present in MRI slice-stacks: In-plane slices
contain high-resolution content, while the orthogonal
planes containing the slice-selection direction are of
low anisotropic resolution. The method relies on the
powerful assumption of self-similarity of local image
structures across these two scales. By training a pair
of dicitonaries to capture the relationship between the
scales, the resolution LR image planes can subsequently
be increased by application of the dictionaries.
|
16:24 |
0409.
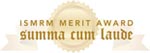 |
Is your system calibrated?
MRI gradient system calibration for pre-clinical imaging 
James Martin O'Callaghan1, Jack Wells1,
Simon Richardson1, Holly Holmes1,
Yichao Yu1, Bernard Siow1,2, and
Mark Lythgoe1
1Centre for Advanced Biomedical Imaging, UCL,
London, UK, United Kingdom, 2Centre
for Medical Image Computing, UCL, UK, United Kingdom
In this study, we present a gradient calibration
protocol, together with an open source phantom, for the
correction of errors caused by imaging gradients that
can be easily implemented in pre-clinical MRI
environments. Mean gradient scaling errors were reduced
from 2.7% to 0.3% through a system calibration which may
be significant in studies of murine brain volumetrics.
Displacements of greater than 100µm were detected in
imaging regions affected by gradient non-linearity and
corrected through a post processing correction.
|
16:36 |
0410.
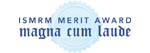 |
A Fast Algorithm for Rank
and Edge Constrained Denoising of Magnitude
Diffusion-Weighted Images 
Fan Lam1,2, Ding Liu1,2, Zhuang
Song3,4, Michael W Weiner3,4,
Norbert Schuff3,4, and Zhi-Pei Liang1,2
1Electrical and Computer Engineering,
University of Illinois at Urbana-Champaign, Urbana,
Illinois, United States, 2Beckman
Institute, University of Illinois at Urbana-Champaign,
Urbana, Illinois, United States, 3Department
of Veteran Affairs Medical Center, San Francisco,
California, United States,4Radiology and
Biomedical Imaging, University of California, San
Francisco, California, United States
We developed a new fast algorithm for joint rank and
edge constrained denoising of 3D magnitude
diffusion-weighted image sequences by extending a
recently proposed majorize-minimize framework for
statistical estimation with noncentral χ distributions.
Specifically, we extended the framework to consider
joint rank and edge constraints, deriving a new
algorithm that decomposes the original noncentral χ
denoising problem into a series of rank and edge
constrained Gaussian denoising problems. We show that
the proposed algorithm achieves similar or even better
denoising performance compared to a previously proposed
algorithm, both qualitatively and quantitatively, but in
significantly less time.
|
16:48 |
0411. |
Automated Organ Detection
in Water-Fat Separated Magnetic Resonance Imaging 
Thomas Demarcy1, Axel Saalbach2,
and Julien Sénégas2
1Ecole des Mines, Saint-Etienne, France, 2Philips
Research Laboratories, Hamburg, Germany
To cope with today’s healthcare challenges of providing
cost-effective care with constant quality, advanced
image analysis techniques that automatically extract
anatomical information from complex, large image
datasets are required. In this work, computer vision
techniques based on trained classifiers and Haar-like
features were extended to 3D and applied to
automatically detect and localize a number of target
organs in 3D water-fat separated, whole-body MR images.
The benefit of using the joint information provided by
water and fat separation was investigated.
|
17:00 |
0412.
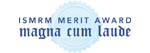 |
High Temporal Resolution
Aortic Input Function Extraction from DCE-MRI Datasets Using
Temporally Neighboring K-Space Samples 
Umit Yoruk1,2, Manojkumar Saranathan2,
Andreas M Loening2, Brian A Hargreaves2,
and Shreyas S Vasanawala2
1Electrical Engineering, Stanford University,
Stanford, CA, United States, 2Radiology,
Stanford University, Stanford, CA, United States
Accurate aortic input function (AIF) measurement is
essential for DCE-MRI applications. We present a method
that estimates the AIF from groups of temporally
neighboring k-space samples, which have better temporal
resolution and temporal footprint than the full k-space
data. Since intensity changes of a structure over time
are reflected in intensity changes of its frequency
components, a portion of k-space too small to form good
images can still be used to quantitatively determine AIF
dynamics. We demonstrated the feasibility of this new
method on a pediatric MR Urography subject and validated
the results using a high spatiotemporal resolution
digital phantom.
|
17:12 |
0413. |
Regression based Pseudo-CT
Creation from Multimodal Images for PET Attenuation
Correction in Hybrid PET-MRI
-
permission withheld
Yaniv Gal1, Sze-Liang Chan1,
Rosalind Lindy Jeffree2, Michael Fay1,2,
Paul Thomas1,2, Stuart Crozier1,
and Zhengyi Yang1
1University of Queensland, Brisbane,
Queensland, Australia, 2Royal
Brisbane & Women’s Hospital, Brisbane, Queensland,
Australia
Inadequate photon attenuation correction (AC) in
Positron Emission Tomography (PET) has serious
implications, such as inaccurate cancer staging or
failure to detect tumours. In hybrid PET-MRI, however,
attenuation has to be corrected in the absence of CT.
MRI-based attenuation correction AC (MRAC) is
challenging because there is no direct correspondence
between photon attenuation coefficient and image
intensity in conventional MRI. We present a regression
based, subject specific, MRAC for PET-MRI using the raw
PET image without AC and multiple MR images, including
structural MRI, pre- and post-contrast dynamic contract
enhanced MRI, all of which are clinically available for
most oncology patients.
|
17:24 |
0414. |
Automated Arterial Input
Function Detection in Ascending Aorta for Breast DCE-MRI
-
permission withheld
Venkata Veerendranadh Chebrolu1, Dattesh D
Shanbhag1, Sheshadri Thiruvenkadam1,
Sandeep Kaushik1, Uday Patil1,
Patrice Hervo2, Sandeep N Gupta3,
and Rakesh Mullick4
1Medical Image Analysis Lab, GE Global
Research, Bangalore, Karnataka, India, 2GE
Healthcare, Buc, France, 3Biomedical
Image Processing Lab, GE Global Research, Niskayuna, NY,
United States, 4Diagnostics
and Biomedical Technologies, GE Global Research,
Bangalore, Karnataka, India
DCE-MRI and pharmacokinetic (pK) model parameters
derived from the DCE data have been commonly used for
characterizing tumor vascular properties quantitatively.
The accuracy of pK parameters depends on the choice of
arterial input function (AIF). Partial volume effects
due to horizontal course of the axillary artery in the
axial plane, motion from pulsation and breathing may
reduce the accuracy of the AIF selected from the
axillary arteries. In this work we demonstrate a
completely automated method for detection of AIF in the
ascending aorta for breast DCE MRI and compare the
results with AIF manually selected by an experienced
radiologist.
|
17:36 |
0415. |
Improved Dual White Matter
and CSF Suppression using MP-nRAGE 
Andrew L Alexander1 and
Steven R Kecskemeti1
1Waisman Center, University of Wisconsin,
Madison, WI, United States
Double inversion recovery (DIR) methods are used to
generate gray matter (GM) specific images by
simultaneously nulling the signals from CSF and white
matter (WM). By using a new, efficient MP-nRAGE method,
it is possible to generate an entire set of whole-brain,
high-resolution, 3D inversion recovery contrast images
with tissues nulled in different frames. By taking the
ratio of the minimum intensity projection to the maximum
intensity projection images of the CSF and WM null
frames, it is possible to generate high quality GM maps
with minimal coil sensitivity effects.
|
17:48 |
0416. |
Model-Free Spectral Fat
Analysis Based on Ultra-Dense Echo Sampling Using a Singular
Value Decomposition Matrix Pencil Method 
Xeni Deligianni1 and
Oliver Bieri1
1Department of Radiology, Division of
Radiological Physics, University of Basel Hospital,
Basel, NA, Switzerland
Assessing tissue fat fraction and composition is of
increased clinical interest as a biomarker for various
muscle and liver diseases. Here, we propose a new method
to achieve an ultra-dense echo sampling in the tens of
microseconds regime within clinically feasible scan
times. This allows a model-free signal time course
analysis based on a singular value decomposition matrix
pencil method to resolve the spectral components of fat.
The effect of the echo spacing and the pre-set of signal
spectral components is analyzed. Four fat peaks are
being resolved and the results are in agreement with
literature. Reducing the preset signal components
results in an underestimation of the fat percentage.
|
|